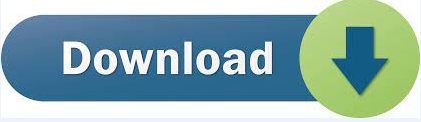
The labels on the x-axis are same as labels on the y-axis. 1: Pearson’s correlation between 13 metrics retained in the model. Hence, pairwise correlation between the metrics is calculated to identify relevant metrics with low correlation.įig. If two features x and y are highly correlated to each other, retaining both the features in the model would be redundant for forecasting, since feature x would provide the same information as feature y.
VMWARE CAPACITY PLANNING BEST PRACTICES SERIES
Threshold setting to flag alerts when the forecasted time series value exceeds the thresholdĪs a first step in the analysis, feature selection was done to identify the important features to incorporate into the model.Forecasting the multivariate time series signal using Vector Autoregressive (VAR) model.Generate multivariate time series from the metrics by sampling them at regular intervals.Variable selection to reduce the total number of variables.The various steps involved in modeling are: Some of the metrics include CPU capacity entitlement, CPU usage, CPU idle summation, disk read, memory overhead, etc. The data was collected across tens of thousands of VMs, for approximately 3 months from January to March, for a total of 78 days. The data collected consisted of a total of 34 metrics averaged over 5 minutes for each VM. The data used for analysis is obtained from vCenter adapter, that collects CPU usage, disk, memory and network related metrics for the virtual machines in the network. The output of the model is a list of VMs which are predicted to fail in the near future. Given a set of metrics to capture the behavior of VMs, the goal is to forecast these metrics and predict ahead when the capacity is going to hit a threshold. In this blog, we discuss the modeling steps involved for VM capacity planning.
VMWARE CAPACITY PLANNING BEST PRACTICES FULL
This helps to take full advantage of the available resources without performance degradation of the VMs. When setting these applications up, it is important to plan the capacity for the VM’s required to run each application. Virtual machines (VMs) are used in many organizations to power many different kinds of business critical applications. This technique can be broadly applied to other areas as well such as monitoring industrial equipment or vehicle engines. In this blog, we will discuss the modeling approaches for forecasting multivariate time series in applications like virtual machine capacity planning. Correlating this with memory and network statistics, as well as SAN I/O and disk I/O metrics, enables the service provider to proactively avoid any bottlenecks and correctly size the VMware Cloud Provider Program platform to avoid performance penalizing or overprovisioning.In the previous blog, a model for forecasting univariate time series with multiple seasonal periods was discussed. CPU Ready Time is the key metric to consider as well as CPU utilization. Employing VMware vRealize Operations™ as a monitoring platform that can trend historical performance data and identify virtual machines with complex or mixed workloads is highly beneficial and its capacity planning functionality assists in determining when to add pCPUs. However, in the world of shared platform and multitenant cloud computing, where this is unlikely to be the case, and the application workload will be unknown, it is critical to not overprovision virtual CPUs, and scale out only when it becomes necessary. So that the vCPU-to-pCPU ratio is optimized and you are able to take full advantage of the benefits of over provisioning, in an ideal world you would first engage in dialog with the consumers and application owners to understand the application’s workload prior to allocating virtual machine resources. This performance impact is further extended as the vSphere ESXi scheduling mechanism prefers to use the same vCPU-to-pCPU mapping to boost performance through CPU caching on the socket. Therefore, while a virtual machine with 4 vCPUs will require the hypervisor to wait for 4 pCPUs to become available, on a particularly busy ESXi host with other virtual machines, this could take significantly longer than if the VM in question only had a single vCPU. While multiple vCPUs are great for workloads that support parallelization, this is counterproductive in the case for applications that do not have built in multi-threaded structures. In the absence of any empirical data, which is generally the case on a heterogeneous cloud platform, it is a good practice, through the use of templates and blueprints, to encourage your service consumers to start with a single vCPU and scale out when it is necessary. The vCPU-to-pCPU ratio to aim to achieve in your design depends upon the application you are virtualizing.
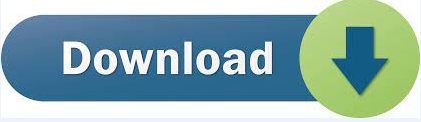